Speech-to-Text (STT) and Speech-to-Speech (STS) technologies are reshaping how we communicate with devices and digital tools. STT, also known as speech recognition, converts spoken words into written text, making it easier to transcribe meetings, lectures, and interviews. Whether you're using Google Docs, a transcription service, or a text app for notetaking, Speech to text AI significantly reduces manual effort.
Meanwhile, STS technology, including Text-to-Speech, enables devices to respond verbally, offering a more dynamic and engaging interaction. This is particularly useful in free speech applications, assistive tools, and smart assistants like Siri, Alexa, and Google Assistant. These systems use microphones and advanced AI to process voice commands, enabling users to search the web, control smart devices, or dictate messages in apps.
From Google Chrome extensions to web apps, Android apps, and text software, the combination of STT and STS is making communication more accessible and efficient. If you're working with audio files or looking for a tool on real-time transcriptions, these technologies will help!
Boosting Productivity with voice typing and Transcription
Voice dictation, powered by Google Speech Recognition and other text technology, allows users to speak directly into the microphone which will then go onto documents, transforming speech into digital text. This text feature enhances productivity by reducing manual typing and making digital tasks more accessible, especially for individuals who find traditional keyboard input challenging.
Beyond dictation, Speech-to-Text (STT) plays a crucial role in transcribing audio content into written form. Whether capturing subtitles for videos, converting recorded meetings into text capabilities, or automating note-taking, this text engine streamlines workflows. Professionals can rely on STT to document data from meetings, call analytics, and lectures with accuracy.
With just a simple start command, STT-enabled tools process audio content efficiently, ensuring seamless documentation and communication.
Enhancing Accessibility through Voice dictation
Speech recognition technology plays a vital role in making digital interactions more inclusive for individuals with disabilities. For those with visual impairments or limited mobility, Speech-to-Text (STT) enables them to navigate devices, control applications, and compose text using only their voice. By eliminating the need for manual typing, STT enhances accessibility and ensures that technology is more user-friendly.
From Education to Entertainment: Versatile Uses of STT and STS
In the education sector, Text-to-Speech (TTS) and Speech-to-Text (STT) technologies help students enhance reading comprehension, practice pronunciation, and engage with learning materials. With voice typing, learners can dictate essays, take notes, and complete assignments hands-free, making studying more efficient. Custom speech solutions also assist language learners by providing pronunciation guidance and reading letters, numbers, and punctuation aloud. These features are accessible across platforms, including Windows 11, where students can activate voice tools via settings and control navigation with a cursor.
In media and entertainment, TTS transforms written content into audiobooks and podcasts, making literature more accessible to auditory learners and those on the go. Businesses utilize custom speech and transcription services to generate voiceovers for advertisements, social media content, and promotional videos. AI-powered tools, such as Azure Speech Services, further enhance time speech processing, ensuring seamless and natural-sounding voice outputs. Whether for education, marketing, or content creation, these technologies provide cost-effective and versatile solutions across industries.
The Future of Customer Service: Automated Solutions with STT and STS
Automated customer service solutions are increasingly leveraging Text-to-Speech (TTS) and Speech-to-Text (STT) technologies to enhance user interactions. Interactive Voice Response (IVR) systems utilize TTS to provide callers with information, assist with troubleshooting, and guide them through processes—all without requiring human intervention.
By integrating speech-to-text for real-time voice typing and transcription, businesses can ensure that customer queries are processed efficiently. This allows for faster responses in multiple languages, including English, improving both accessibility and accuracy. Advanced tools like Dragon speech recognition software further enhance automation, making interactions smoother and more intuitive.
With online STT solutions available for instant deployment and download, companies can optimize their customer support strategies. These AI-driven technologies not only improve typing efficiency for support agents but also help businesses manage resources effectively while maintaining high levels of customer satisfaction. Whether on desktop or mobile, users can simply start a conversation using their voice, creating a seamless and engaging customer experience.
Technical Comparison: Speech to Text vs Speech to Speech AI
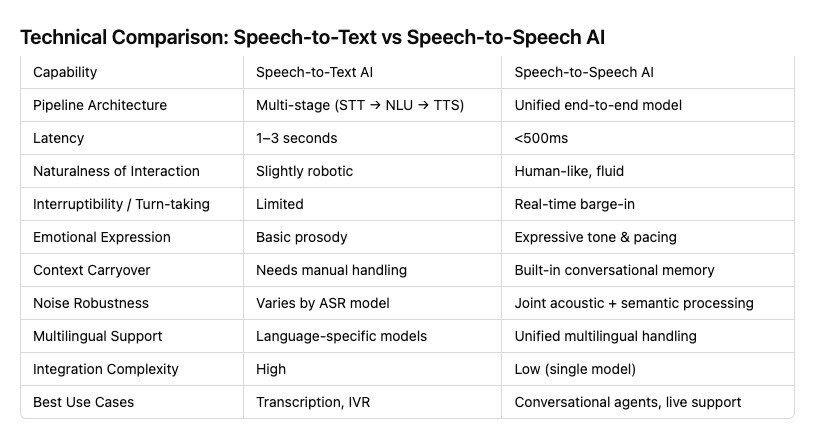
When evaluating voice-based AI solutions, it’s important to understand that not all technologies are created equal. Two prominent categories—Speech-to-Text AI and Speech-to-Speech AI—offer distinct advantages and serve different needs. Below is a closer look at how these two solutions compare across key technical dimensions:
-
Pipeline Architecture
-
Speech-to-Text (STT): Typically follows a two-step approach—first converting speech to text, then applying natural language processing (NLU) before any response is generated.
-
Speech-to-Speech: Streamlines the pipeline by integrating input capture, understanding, and vocal response into a more unified system. This holistic approach helps reduce processing time and complexity.
-
Latency
-
Speech-to-Text: Usually experiences a short but noticeable delay (1–3 seconds) while the system transcribes and processes the speech.
-
Speech-to-Speech: Achieves near real-time performance, often under 500ms, making conversations feel more fluid and natural.
-
Naturalness of Interaction
-
Speech-to-Text: Responses can sound slightly robotic or stilted due to the text-based intermediary step.
-
Speech-to-Speech: Tends to offer more human-like, fluid interaction. The voice output is designed to mimic human intonation and flow.
-
Interruptibility & Turn-Taking
-
Speech-to-Text: Interruptions can be handled, but the system often needs to pause and reprocess inputs, which may disrupt the conversation flow.
-
Speech-to-Speech: Real-time barge-in capabilities allow for smoother turn-taking, so users can interject or correct themselves naturally.
-
Emotional Expression
-
Speech-to-Text: Generally limited to basic prosody—speech is transcribed, then a response is generated that might not convey nuanced emotion.
-
Speech-to-Speech: Can offer expressive tone and pacing, making the output sound more engaging and empathetic.
-
Conversational Context
-
Speech-to-Text: Can vary widely depending on the quality of the Automatic Speech Recognition (ASR) model. Some solutions struggle with context or continuity.
-
Speech-to-Speech: Often integrates robust conversational memory, enabling the AI to handle context shifts more naturally within a continuous spoken interaction.
-
Noise Robustness
-
Speech-to-Text: Performance depends heavily on the ASR model and its training. Some systems falter in noisy environments.
-
Speech-to-Speech: Often incorporates both acoustic and semantic processing to better isolate speech from background noise.
-
Multilingual Support
-
Speech-to-Text: Language-specific models are common, requiring separate setups or modules for each language.
-
Speech-to-Speech: Some solutions offer a unified multilingual platform, seamlessly switching between languages in real time.
-
Integration Complexity
-
Speech-to-Text: Easier to integrate into existing applications that already handle text-based data, such as chatbots or transcription tools.
-
Speech-to-Speech: More complex to implement, as it requires end-to-end voice handling—capturing audio, processing semantics, and generating vocal responses.
-
Best Use Cases
-
Speech-to-Text: Ideal for transcription services, IVR systems that route calls, and basic voice commands where text output or processing is sufficient.
-
Speech-to-Speech: Excels in conversational agents, customer service bots, and any scenario where natural, human-like dialogue is key.
Speech-to-Text (STT) AI vs. Speech-to-Speech (Direct S2S) AI: A Side-by-Side Comparison
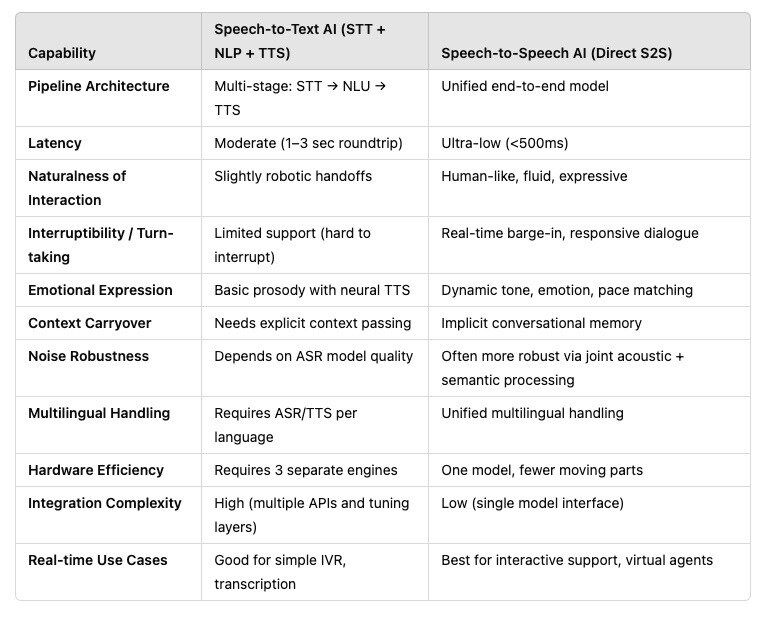
When evaluating conversational AI solutions, one of the most critical decisions is whether to use a Speech-to-Text (STT) approach—where spoken words are first transcribed into text for further processing—or a Speech-to-Speech (S2S) approach, which converts spoken input directly into spoken output in near real time. Below is a concise breakdown of how these two approaches stack up across key dimensions:
-
Pipeline Architecture
-
STT: Follows a multi-stage process: first transcribing speech to text, then applying natural language processing (NLP), and finally converting the output text back to speech (TTS).
-
S2S: Adopts a more unified end-to-end model, capturing and interpreting spoken input directly to produce spoken output. This can reduce complexity and speed up interactions.
-
Latency
-
STT: Often experiences moderate delays—ranging from one to three seconds—due to the multiple steps involved.
-
S2S: Achieves ultra-low latency (often under 500ms), making it highly suitable for real-time conversations.
-
Naturalness of Interaction
-
STT: Can sound slightly robotic, as the TTS engine might produce less expressive speech.
-
S2S: Offers a more fluid, human-like feel. Advanced S2S systems often include expressive vocal nuances, making interactions more engaging.
-
Interruptibility / Turn-Taking
-
STT: Interruption handling can be limited because the system may have to pause and reprocess the audio input.
-
S2S: Real-time barge-in capabilities allow users to interrupt or correct the system, facilitating more natural, dynamic conversations.
-
Emotional Expression
-
STT: Relies on basic prosody provided by standard TTS engines, which may sound monotone or lack nuanced emotion.
-
S2S: Can integrate emotional tone and expression into responses, offering a more empathetic user experience.
-
Context Carryover
-
STT: Context depends on how well the ASR model handles linguistic cues and how effectively the NLP module retains conversation history.
-
S2S: Joint acoustic and semantic processing can enable more robust tracking of conversational context, enhancing continuity.
-
Noise Robustness
-
STT: May require specialized noise-cancellation or training for different acoustic environments.
-
S2S: Often employs combined acoustic and semantic strategies to filter out background noise more effectively.
-
Multilingual Handling
-
STT: Typically needs separate ASR/TTS engines for each language, increasing integration overhead.
-
S2S: Can offer unified multilingual capabilities, automatically handling multiple languages in a single pipeline.
-
Hardware Efficiency
-
STT: Involves multiple APIs and engines (ASR, NLP, TTS), which can demand more computational resources.
-
S2S: Uses one model or a more streamlined setup, reducing hardware requirements and simplifying deployment.
-
Integration Complexity
-
STT: Requires linking multiple layers—ASR, NLP, and TTS—each with its own tuning and maintenance needs.
-
S2S: Streamlined integration, with fewer components to manage and optimize.
-
Best Real Use Cases
-
STT: Ideal for simple IVR systems, transcription services, and scenarios where text output or logs are essential.
-
S2S: Suited for interactive support, virtual agents, and any use case demanding real-time, human-like voice interaction.
Maturity Roadmap Overview
.svg)
This roadmap illustrates a progression toward a fully optimised customer experience over an 18-month span. In the first three months, the focus is on enhancing customer self-service options—reducing friction and allowing customers to find answers on their own. From months four to six, attention shifts to operational efficiency, streamlining processes and refining internal workflows.
By months 12 to 18, the business aims to lead in customer experience, having laid the groundwork to deliver standout, personalised interactions that differentiate it from competitors.
Voice AI: Benefits in Numbers
-2.jpeg?width=704&height=484&name=Image%2031-03-2025%20at%2011.24%20(1)-2.jpeg)
Voice AI technology significantly outperforms traditional customer interaction channels such as legacy IVR systems and chatbots, delivering measurable business benefits:
-
Call Containment: Voice AI assistants effectively manage 50–80% of calls without human intervention, compared to just 10–30% for legacy IVR systems and 20–40% for chatbots.
-
First Contact Resolution (FCR): Voice assistants achieve impressive FCR rates (80–85%), greatly exceeding legacy IVR (20–40%) and chatbots (30–50%).
-
Customer Engagement: Voice AI excels in engagement by providing a natural conversational experience, significantly reducing customer fatigue compared to IVR systems (low engagement due to keypad-based navigation fatigue) and chatbots (medium engagement impacted by text interaction fatigue).
-
Customer Satisfaction (CSAT) Uplift vs Average Handling Time (AHT): Voice AI assistants drive a high uplift in customer satisfaction through natural conversational capabilities, unlike legacy IVRs which prolong interactions, or chatbots that offer a moderate improvement of around 2–5%.
-
Live Agent Escalations: Voice AI dramatically reduces escalation rates to live agents to less than 30%, in stark contrast to the prolonged escalation processes common with legacy IVR systems, and the moderate escalation rates associated with chatbots.
In summary, voice AI assistants offer compelling operational advantages by enhancing customer interactions, significantly increasing resolution efficiency, and elevating overall user satisfaction.
No Comments Yet
Let us know what you think